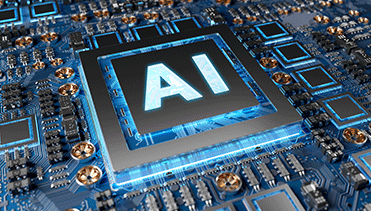
We aim to advance modern computer systems based on specialized hardware in the post-Moore’s law era. We conduct research in various fields of hardware design such as computer architecture, VLSI, FPGA, hardware/software co-design, and processing-in-memory with holistic design approach to improve overall system performance. Our current mission is to build a high-performance and scalable computing platform for future AI applications.
Please see the following selected publications to learn more about CastLab’s research.